This is the biometric age with biometric methods of identification soaring in popularity.
Today, almost every modern smart mobile device features fingerprint scanners and voice and facial recognition as the default mechanisms for access control.
As their adoption grows, their application is also expanding in scope to enable features such as app purchase and in-app payment, e-wallets and access to password managers.
From an IT systems administration’s perspective, the advantage of these methods of identification over passwords, even one-time passwords (OTP) and two-factor authentication (2FA), is the great difficulty of faking a biometric sample or manipulating the biometric sensor into producing a false-positive result.
For many years, this last statement stood largely unchallenged. However, at this moment, amidst the proliferation of biometric applications in the consumer market (and specifically mobile devices and IoT), we are possibly looking at the tipping point for the security of biometric applications.
This tipping point centres around two major concerns surrounding biometric identification methods:
#1. Are biometric methods of identification as secure as we assume they are?
#2. What new risks do we face in the short and long term with regard to privacy and the risk of irreversible identity theft?
The Maker/Hacker Paradigm In Biometric Applications
The recent news about the Samsung Galaxy 10S+ fingerprint hack is extremely interesting to contemplate in relation to this tipping point.
In this social media event, a researcher 3D-printed a resin mold of his own fingerprint. He then demonstrated via image sharing website Imgur how that resin mold could be used to unlock his own Samsung Galaxy 10S+ device.
We need to treat this finding with a certain level of caution. In the video demo it appears as if he was unlocking the device with the resin mold while wearing a glove—and yet he is holding it with his index finger. One might assume that this is the same finger used to unlock the device.
The initial impression is that the researcher was able to crack an industry-grade fingerprint reader using commoditised technologies (cell phone camera, commercially available 3D printer and software).
To assess if that is indeed true, it is important to understand how the Qualcomm Snapdragon Sense ID Fingerprint Sensor (which is used by the Samsung Galaxy 10S+ device, among other devices) works.
Not All Biometric Methods Are Created Alike
Ascertaining a user’s identity using a fingerprint involves several steps. One must collect the sample, remove the noise (such as dirt and blur from miniscule movement), extract the key features and calculate the match to the baseline sample. There are also controls around the detection process to ensure that the reader is scanning a real, living human finger.
Despite the common misconception that fingerprints are matched by checking the overlap or visual similarity of two images, modern algorithms do not actually work this way. Algorithms are based on mathematical models that identify families of features (such as ridges, lines and gaps) and build a mathematical expression of these features; for example, the distance between the centre of one shape pattern and a distinctive line. This probabilistic approach allows for a much faster and more accurate calculation, while also avoiding the need to retain an image of the original sample on the device, thus preserving the user’s privacy.
When it comes to fingerprint readers, there are a few basic methods for performing the first step of collecting the sample. The methods include: optical, capacitive, ultrasound, e-field, electro optical, pressure sensitive, thermal, and MEMS (microelectromechanical systems).
Of these, three are commonly used in modern smart phones: capacitive, optic and ultrasonic.
In the capacitive method, the fingerprint surface is scanned onto a 2D grid of a conductive sensor that detects the minute electric capacity differences caused by the skin folds that make up the ridges and valleys of a fingerprint.
In the optic method, a 2D image of the creases and folds of the surface is collected by detecting the absorption, scattering and reemitting of a source of illumination (such as LED or laser).
In the ultrasonic method, a 3D image of the fingerprint is sampled by measuring the variant of the echo produced by the skin folds of a fingerprint.
The fingerprint sensor that Samsung uses relies on the last method. According to Qualcomm, this method has an advantage over other methods as it can scan deeper and produce more accurate results in creating a 3D image compared to the 2D image rendered in other methods. Additionally, it features a built-in liveness detection algorithm.
According to official documentation, the scanning supports material penetration technology that allows the sensor to scan through glass, plastic, aluminum and more. It could be this depth that is the culprit in this case — the researcher may have used the same index finder to press down the resin mold. Naturally, this would also defeat the liveness detection mechanism.
Should We Run For Cover?
This maker/hacker approach to circumventing biometric identification is nothing new. A hacker by the name Jan Krissler demonstrated over four years ago how he could fake the fingerprints of the German defense minister, Ursula von der Leyen, using a photograph released by her own PR office.
Knowing this, should we be concerned? The simple fact that it is feasible doesn’t necessarily make it probable. For this risk to become a significant concern, the attack surface must change and evolve into a scalable vector that can be automated, at least to a certain degree. In other words, as long as the biometric application is paired with a specific physical device that the attacker must have physical access to, the likelihood of this threat affecting the general public is relatively low. We can still expect to see weaknesses published by researchers whose main motivation is pulling off a technical conquest and attaining recognition as opposed to the cybercriminal and hacker counterparts who are driven by profit and the quest to monetise the weaknesses they uncover.
Regardless of the validity of this specific experiment, this episode is an excellent example of the inherent tradeoff between the convenience of not having to type passwords and the risk of having an authentication/authorisation mechanism lacking a revocation mechanism.
The Future Of Biometric Identification
We can expect biometric identification to continue to grow in popularity and expand in application scope. Hot trends, including IoT applications for smart homes, e-voting, and biometric border controls, will intensify interest among the hacking community to make attempts.
To overcome these risks, we need to consider adding additional layers of anti-fraud or business intelligence where applicable (that is, identify suspicious/abnormal application activity, use GPS to correlate identification events, and so on) to the applications using biometric identification to reduce the risk of misuse or fraud. In this way, we can make it as difficult as possible for malicious agents to complete fraudulent transactions.
We have yet to reach the tipping point in which the potential weaknesses in biometric applications of identification/authentication are a major concern. I would argue that compared to passwords, biometric identification is still a much more secure method, despite its inherent flaw of lacking the revocation mechanism.
However, while we are not at the tipping point yet, we should be sensitive when applying biometric solutions that might drive us to that tipping point.
There are alternatives to the more traditional biometric solutions (for example fingerprints, retina and voice recognition) such as cognitive and behaviouristic methods that could offer a good balance between with level of security and the revocation/natural decay of the baseline as people’s cognitive abilities and behaviours gradually change over time.
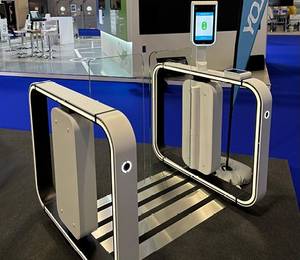
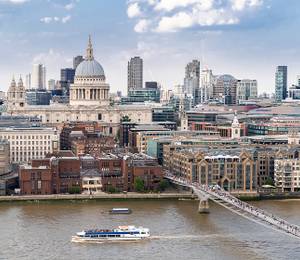
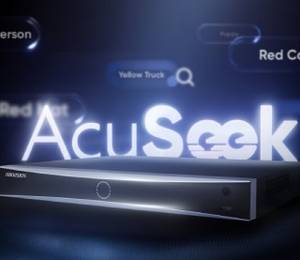
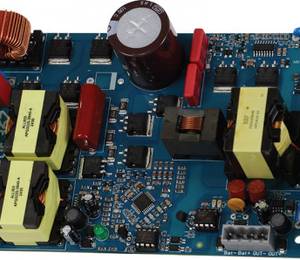
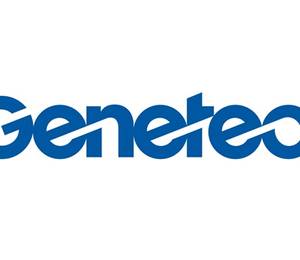
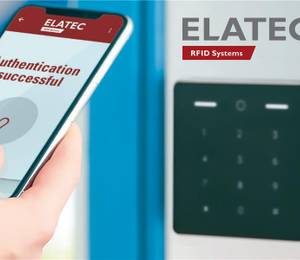
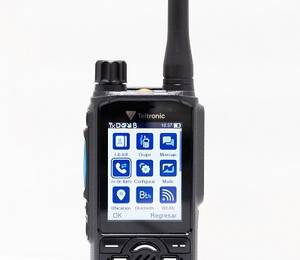
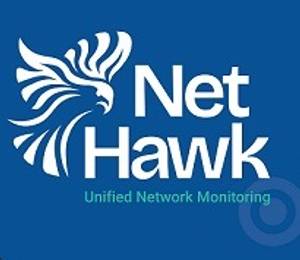
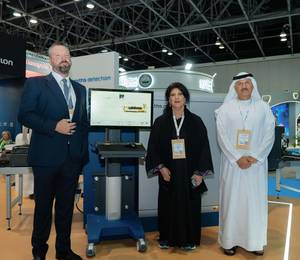